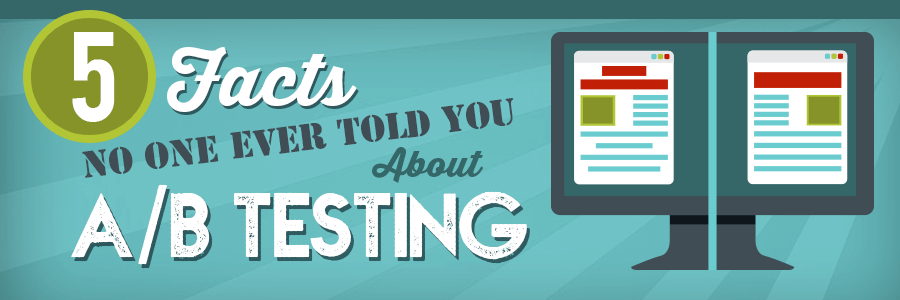
5 Facts No One Ever Told You About A/B Testing
Table of Contents
A/B testing gives you insight into what works best for your email marketing campaigns. However, lots of A/B tests generate flawed data that lead to poor strategic decisions. Usually, this comes from a misunderstanding of how to correctly begin-and end- an A/B test. These five A/B testing facts will help you understand how A/B testing works and where you can improve your own testing process.
A/B Testing is Only as Good as the Underlying Assumptions
Let’s say I want to test a call-to-action (CTA) button. Right now, the color is red. My assumption is that if I change the color of the button to blue, I will see a 20% increase in clicks.
This is a common testing scenario. When blue results in a 20% lift, a marketer will claim victory. When the blue does not meet the 20% lift criteria, or just barely improves over the red CTA, a marketer will claim failure.
But let’s back track a moment. Why is red currently the CTA button color? Why is blue the variation being tested? Is there a practical business reason to run this test, or is it purely for experimentation? Why not a yellow button? A green button?
There are thousands of testing variations available just with a simple CTA button. Since you can’t test every variation, it's best to have a justification for testing before the test starts.
Without good testing assumptions, you’re simply generating data for the sake of generating data. This never leads to a positive outcome, unless you count more data to analyze as core metric.
Before committing time and resources to a test, ask yourself:
- Why am I performing a test?
- What is the justification for choosing this variation (B version over another) ?
The best A/B testing results occur when you have:
- An expert understanding of the business problem.
- Solid split testing ideas to correct the business problem.
Research and experience will lead to good assumptions which leads to data that can actually be used beyond one split test.
You Need to A/A Test First
Before jumping into an A/B test experiment, you need to conduct an A/A test first.
Most marketers will look take a broad overview of their metrics before deciding on what and how to A/B test.
Unfortunately, the interpretation of all available data within a certain period time is not like the data you obtain from an A/B test. A split test requires sampling your subscriber list at random, or some other convenience method like segmenting your subscriber database.
The idea is that a smaller piece of the pie represents the way the whole pie tastes. However, the customer experience is usually a complex, unpredictable journey.
Customers check their inbox at different times, with noise going off in the background, with other tabs open on other devices all while multitasking work, family and life.
Here’s a very practical example as to why you need to A/A test first.
Now, let’s create a 50/50 split with our 300 subscribers. Half will obtain variation A and half will obtain variation A. We won’t be looking at a big metric like CTR. We just want to know if the subscriber clicked or not.
We will use this formatting to keep it easy:
0=customer did not click
1=customer did click
Now, let’s look at how the other 50% performed.
There is clearly a large difference in clicks. The two groups received the same email but responded much differently. Total clicks in the second group is much higher. Many would expect both groups to have roughly the same amount of total clicks. More often than not, there is so measurable variation between subscribers receiving the same email.
A/A tests will establish a baseline for your A/B tests. The goal becomes a question of how much lift you can get from improving on the results from your A/A test, not just from giving subscribers a different variation.
Often, A/A test results look like this.
A 26% difference (405 clicks vs 322 clicks) means you’ll need to pick a B variation that performs better than the natural difference between the A groups.
Without first doing an A/A test, you may have performed an A/B test that only resulted in a 10% lift. While that’s great in isolation, it actually underperforms in comparison to the A/A test. The key takeaway here is that an improvement can't be measured until the existing variation within your subscribers is measured first.
Open Rates Need to Be Similar Across A/B Testing Groups for Data to be Valid
The ultimate goal of an A/B test is to let your customers tell you what they like best so you can put it into motion across all your marketing efforts.
When conducting an A/B test, if the open rates aren’t the same between the groups, you need to discard the test (unless you’re testing subject lines only).
Let’s say you’re interested in testing a promotional coupon inside of an email. Group A receives this.
Group B receives this.
The initial results show a 70% conversion rate from variation A and a 52% conversion rate from variation B.
But data always tells a deeper story. All you have to do is ask.
The differences in open rates are problematic. We can’t actually declare variation A the winner of the experiment. We would have to run the test again and hope for a better open rate to obtain valid data.
Every time something goes wrong, the power of your test results decreases. Practically speaking, this means you need to be less confident in your results and less confident in how you may apply any intelligence gained.
When in doubt, scrap everything and re-test.
Your A/B Testing Results May Not Be from Testing at All
In statistics, there is the concept of a random error. The random error is everything your test didn't include that is still impacting the outcome. Randomness works behind the scenes to confuse the data analysis process even more.
While you are performing A/B tests, keep the concept of randomness in mind. There are always other forces acting on your customers.
Sure, It’s nice to see a 300% conversion rate increase from changing a subject line, but it’s not actually 300% due to the subject line. A/B testing only records a certain customer touch point, not the entire experience, making revenue attribution modeling very difficult. Customers interact with 11 brands per day through email, not to mention all of the other ads they see and conversations they have about various products and services. This is important to remember when evaluating your A/B testing process.
Picking winning variations is essential to crafting better email marketing campaigns. By being realistic about the impact of your A/B testing, your treating data with caution. This approach isn't as fun as wild increases in metrics but will lead to a more focused marketing campaign strategy.
Crafting winning campaigns is a team sport!
You’ll Get Different Results at Different Periods in Time
There’s nothing more damaging to split testing data than stopping the experiment too early.
No matter how long you choose to run the A/B test for, you’ll most likely get different results at different points in time.
Look at this A/B example of a newsletter sent out to 2000 subscribers (1000 received variation A and 1000 received variation B). Total clicks on the newsletter were tracked for 7 days.
Days 1 through 3 and days 1 through 5 both declare B the winner. However, by day 7, A is the clear winner.
The business included offers redeemable for 7 days in the newsletter, so it wouldn’t have made sense to stop tracking clicks at any time prior to day 7.
Generally, testing something for 30 days is ideal. However, the business objective needs set the testing start date and end date. You need to let the customer journey play out for long enough so that you're comfortable with saying, "this data reflects our entire customer base."
Embrace Careful Data Analysis
Even when A/B testing results in a clear winner, you should always be skeptical about your data. Statistics can be a dirty process. A small misinterpretation of split testing data can have a big impact across your email marketing campaigns.
In an ideal marketing world, you wouldn’t have to split test anything. You would just know what works and for how long. A/B testing helps you get closer to that goal, especially when you aim for marketing results, not tesing results. Only test what you can justify and have a data backed assumption in mind.
A/B testing is one of the many valuable research tools available to email marketers. By taking your results with a grain of salt and continuing to fine tune the testing process, you'll be on a path to greater data centered email marketing campaigns.
[Tweet “5 Facts No One Ever Told You About A/B Testing”]
Pinpointe Newsletter
Join the newsletter to receive the latest updates in your inbox.